The Consortium of Molecular Design at BYU provides cutting edge interdisciplinary research opportunities for students to push the envelope for protein engineering and drug discovery.
We use close collaboration between laboratories at BYU in Physics, Chemistry, Computer Science, LifeSciences, and Engineering to tackle these challenging topics from all angles.
We actively seek industrial collaboration and support for our efforts and are excited to explore mutually beneficial application of all state-of-the-art technologies to revolutionize molecular design.
News and Events
Selected Publications
Research Opportunities
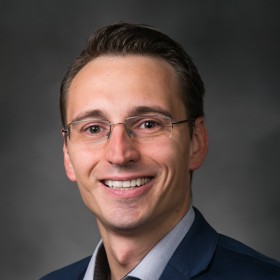
- ProSPr - Protein Structure Prediction
A cross divisional team of physicists, computer scientists, biologists and chemists implements a novel protein structure prediction pipeline to solve one of the oldest challenges in computational biophysics: The Protein Folding Problem.
We will apply our pipeline to a global community wide blind test in 2020 called CASP14.
The work entails:
- training of convolutional neural networks
- design of simulation algorithms
- high performance super computer usage
- chemical and biological evaluation of results
- Radical SAM Engineering
Together with the Chemistry department at BYU, we are developing algorithms that aid the systematic design of novel enzymes.
These enzymes can be applied to a variety of use cases, such as fertilizer production, detergent production, or drug production.